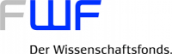
FWF HOnEst
Ontologies represent and capture both terminological and assertional knowledge underlying novel intelligent systems including question answering systems (e.g., IBM Watson) or conversational agents (e.g., chatbots). Increasingly, such systems make use of very large, automatically extracted ontologies which often contain defects introduced through the extraction process. These defects hamper the system’s ability to provide factually correct and unbiased answers.
The ontology evaluation community has proposed a range of methods for the automatic verification of ontologies but these methods cannot verify aspects that require human involvement, e.g., correctness with respect to common sense knowledge. Research on human-centric ontology evaluation that could address such quality aspects has, so far, neither reached a good characterization of the problem nor provided scalable methods for addressing it. Human Computation (HC) techniques could be a promising solution to human-centric ontology evaluation, yet, their applicability to conceptual structures such as ontologies as opposed to cognitively less complex data (e.g., text, images) raises several open issues.
HOnEst fills these gaps by investigating the following research questions:
- (RQ1) Which ontology evaluation tasks can be (currently) only solved with human involvement?
- (RQ2) How to solve these evaluation tasks successfully with HC techniques?
- (RQ3) How to scale HC for the evaluation of very large ontologies?
HOnEst will strengthen the human-centric evaluation area within the ontology evaluation research with novel contributions such as:
- a systematically-classified catalogue of human-centric ontology evaluation tasks (HET) identified partly through a Systematic Literature Review (RQ1)
- the VeriCoM2 HC-based approach for solving HET, developed with a Design Science method (RQ2)
- evidence-based guidelines for optimal HC-configurations
- the first benchmark for (human-centric) ontology evaluation
Within HC, HOnEst will focalize research on the nascent topic of conceptual model evaluation, which was investigated across diverse communities. To that end, it will address challenges such as (1) selecting suitable model fragments to be used as context within HC tasks and (2) measuring (dis)agreement of diverse viewpoints collected through HC.
A novelty for both fields is investigating scalability aspects of human-centric ontology evaluation (RQ3) by creating hybrid human-machine systems that combine human and computational components (e.g., machine learning classifiers) alike. The approach will be tested in two use cases: (1) evaluating a large-scale ontology of research topics and (2) evaluating the WebIsALOD knowledge graph focusing on tail (i.e., not mainstream) entities.
Partners
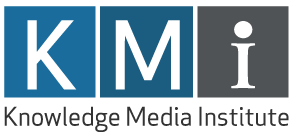
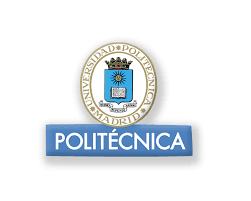